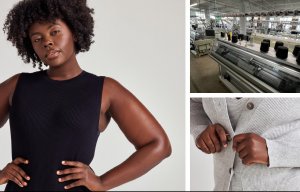
Launch of Losano Wholegarment seamless collection
Opinion
Unique combination of machine learning for automated style training and novel algorithms for defect detection.
10th January 2023
Adrian Wilson
|
Leicester, United Kingdom
Over the past three years, a dedicated AI development team at Shelton Vision, based in Leicester, UK, has been developing tailored machine learning solutions for the textiles industry.
The aim has been to elevate the detection process and the accuracy of naming and grading subtle defects in textiles, in real time within production environments.
“Big Data ‘off-the-shelf’ systems such as those behind technolgies like facial recognition and Google Maps involve reading many thousands of single images each second and simply take too long to accumulate sufficient data for what’s required in this specific case,” says Shelton Vision CEO and managing director Mark Shelton. “A feature of the textile industry is that in many sectors, the product range changes several times within a year and it is not uncommon to have to inspect hundreds, if not thousands of different styles in a year based on precise settings.”
In terms of defect types, he adds, there may typically be over 100 that need to be accurately detected, classified (named) and graded in real time.
“Added to this is the need to ‘filter out’ the random occurrence of ‘non defects’, such as loose threads, lint and dust on the surface – the number of which can be higher than actual defects – and it is clear that a bespoke system is required.”
Metadata
The development team has consequently established metadata for identifying defect properties, enabling the successful identification of faults from a much smaller number of images.
“The system employs a unique combination of machine learning for automated style training and novel algorithms for defect detection, to provide high quality images for the AI real time defect classification and grading software,” Shelton explains. “Due to the inherent variation in fabric features – raw materials, construction, texture, colour and finishes, as well as the differing product quality standards in value chains and the regional variations in what defects are called – our AI engine uses models built for each individual company or group of companies, or product value chain.”
The AI models are constructed so that the user operatives can populate them with their own data produced by the vision system or by obtaining defect images from another imaging source (eg a mobile phone camera).
The occurrence of defects is sporadic and many defect types occur infrequently, although when they do, they can have severe consequences. These scenarios reinforce the need for the AI engine to be quickly set up and able to operate accurately with limited data sets of typically between 30 and 50 good quality images per defect type.
A further feature is a tool enabling the user to periodically ‘clean up’ the AI data during the set up phase. This is used to resolve conflicting data and to correct mis-named images.
Process operation
Generally, the highest cost component of fabric production is the raw material and in addition to finished product inspection, a cost effective use for vision systems is in process operation.
“There is a need for the real time detection of defects that are being created in separate processes, such as printing or coating and for real time automated systems that can accurately determine the defects and their severity and provide a reliable signal for an operative to rectify the issue, This can result in considerable savings.
Prior to Shelton introducing powerful customised machine vision and real time defect classification, the only systems available were those that required manual sifting through vast numbers of images, which included both real defects and ‘non defect’ images. The task was very often overwhelming and did not provide much benefit beyond manual fabric inspection.
The Shelton Vision AI development has been completely self-funded and there are many systems already operating in the field in demanding textile applications, as business critical systems that require robust design to provide the needed up time.
“Studies show that the intelligent fabric inspection systems of Shelton Vision’s WebSpector with tailored AI solutions out-perform human inspection in terms of speed, accuracy and consistency,” says Jason Kent, CEO of the British Textile Machinery Association (BTMA). “In addition, there is a global drift of people away from the type of activity that fabric inspection requires, making the company’s technology increasingly valuable to forward-looking mills.”
Business intelligence for the fibre, textiles and apparel industries: technologies, innovations, markets, investments, trade policy, sourcing, strategy...
Find out more